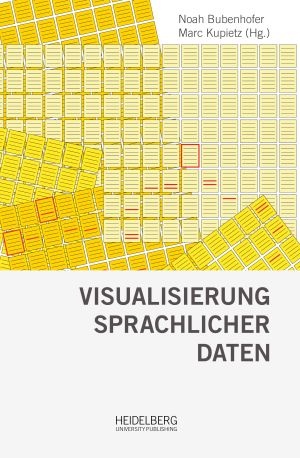
Zitationsvorschlag
Lizenz

Dieses Werk steht unter der Lizenz Creative Commons Namensnennung - Weitergabe unter gleichen Bedingungen 4.0 International.
Identifier
Veröffentlicht
Visualisierung sprachlicher Daten
Visual Linguistics – Praxis – Tools
Visualisierungen spielen in den Wissenschaften eine wichtige Rolle im Forschungsprozess. Sie dienen der Illustration von gewonnener Erkenntnis, aber auch als eigenständiges Mittel der Erkenntnisgewinnung.
Auch in der Linguistik sind solche Visualisierungen bedeutend. Beispielsweise in Form von Karten, Baumgraphen und Begriffsnetzen. Bei korpuslinguistischen Methoden sind explorative Visualisierungen oft ein wichtiges Mittel, um die Daten überblickbar und interpretierbar zu machen.
Das Buch reflektiert die theoretischen Grundlagen wissenschaftlicher Visualisierungen in der Linguistik, zeigt Praxisbeispiele und stellt auch Visualisierungswerkzeuge vor.
----
Die Forschungsdaten zum Beitrag von Wolfer/Hansen-Morath: "Visualisierung sprachlicher Daten mit R" sind abrufbar unter https://doi.org/10.11588/data/6SO6TG.